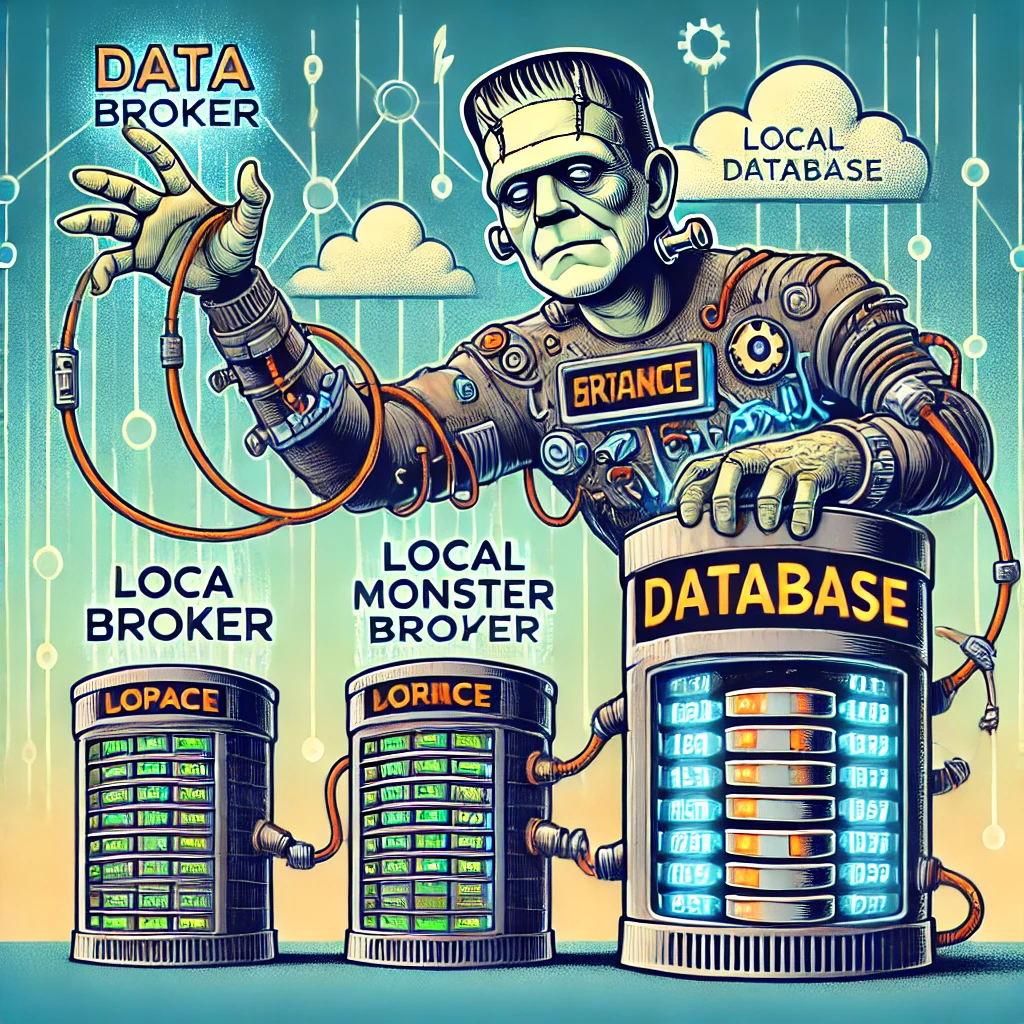
Here’s a straightforward example of how data replication can be achieved using the Frankenstein Automation-Gateway.com to transfer data from a remote broker to a local MonsterMQ Broker.
The local MonsterMQ Broker is configured so that data is stored in TimescaleDB to maintain a historical record. This process converts the current state of the UNS into archived historical data.
It will also create a Frankenstein OPC UA Server, allowing you to access the data from the MQTT broker. However, since we have it data-agnostic, all the data in the OPC UA Server will be available as a string data type.
Monster.yaml
Create a file monster.yaml with this content :
TCP: 1883
WS: 1884
SSL: false
MaxMessageSizeKb: 64
QueuedMessagesEnabled: false
SessionStoreType: POSTGRES
RetainedStoreType: POSTGRES
ArchiveGroups:
- Name: "source"
Enabled: true
TopicFilter: [ "source/#" ]
RetainedOnly: false
LastValType: NONE
ArchiveType: POSTGRES
Postgres:
Url: jdbc:postgresql://timescale:5432/postgres
User: system
Pass: manager
Frankenstein.yaml
Create a file frankenstein.yml with this content and adapt the Host of the soruce broker and the Topic paths which you want to replicate from the source to your local MonsterMQ Broker.
Servers:
OpcUa:
- Id: "opcsrv"
Port: 4840
EndpointAddresses:
- linux0 # Change this to your hostname!
Topics:
- Topic: mqtt/source/path/Enterprise/Dallas/#
Drivers:
Mqtt:
- Id: "source"
Enabled: true
LogLevel: INFO
Host: test.monstermq.com # Change this to your source MQTT Broker!
Port: 1883
Format: Raw
Loggers:
Mqtt:
- Id: "source"
Enabled: true
LogLevel: INFO
Host: 172.17.0.1
Port: 1883
Format: Raw
BulkMessages: false
LogLevel: INFO
Logging:
- Topic: mqtt/source/path/Enterprise/Dallas/#
Docker Compose
Create a docker-compose.yaml file with this content and then start it with docker-compose up -d
services:
timescale:
image: timescale/timescaledb:latest-pg16
container_name: timescale
restart: unless-stopped
ports:
- "5432:5432"
volumes:
- timescale_data:/var/lib/postgresql/data
environment:
POSTGRES_USER: system
POSTGRES_PASSWORD: manager
monstermq:
image: rocworks/monstermq:latest
container_name: monstermq
restart: unless-stopped
ports:
- 1883:1883
- 1884:1884
volumes:
- ./log:/app/log
- ./monster.yaml:/app/config.yaml
command: ["+cluster", "-log FINE"]
frankenstein:
image: rocworks/automation-gateway:1.37.1
container_name: frankenstein
restart: always
ports:
- 1885:1883
- 4840:4840
environment:
JAVA_OPTS: '-Xmx1024m'
volumes:
- ./frankenstein.yaml:/app/config.yaml
- ./security:/app/security
volumes:
timescale_data: